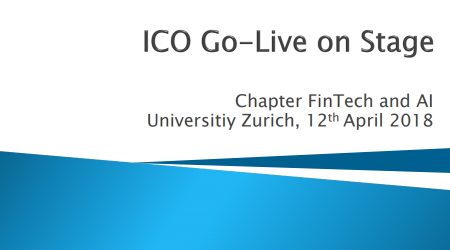
FinTech in light of fast evolving technologies
April 12, 2018 | Case Study
April 12, 2018 | Case Study
June 18, 2015 | Publication
Data lineage should be an automated process and added to the “Business As Usual” operations. However, it has proven to be a task not so easily accomplished and not without its problems.
The team must manually confirm that the lineage is complete and address any gaps by going into the codebase and determining the proper stitching.
The Natural Lineage Processing can identify gaps where more code is expected. It can suggest likely connections by analyzing the lineage and recognizing similar paths.
Data lineage may be complete and accurate, but only accessible at a very technical level. The business needs to understand the data flows but cannot make sense of the large, highly-connected, and technical lineage.
A complete inventory of the physical data elements is compiled and a large effort is put together to create a complete data dictionary. An army of experts are asked to map the physical data elements to the concepts defined in the data dictionary schema.
Data properties, data statistics, and data flows feed into the Natural Lineage Processing engine which groups similar physical elements. These groups are checked with a smaller number of subject matter experts whose feedback is used to refine the groupings in a fast, iterative process.
The business needs to understand the quality of the underlying data, not just the flows. But How do you ensure that the data is of high quality? How would your QA process catch high-quality data that is used incorrectly?
Teams of statisticians, analysts, and data scientists will crawl through databases manually or develop custom scripts to measure data properties. These processes are difficult to maintain and incomplete.
The Natural Lineage Processing toolkit can automate the data quality assessment. It includes standard statistical testing, but algorithmically determines the correct metrics based off of the inherent data properties coupled with the contextual information from the data lineage.
Detecting and preventing fraud is a target for the business…. But how do you find fraud?
Subject matter experts set thresholds or develop tailored models for the specific fraud channel. Some rudimentary ML models may be developed.
More complex models are developed with little-to-no input required from subject matter experts. Data Lineage information is also fed into the models to capture more complex expressions of fraud.
Data Lineage is often utilized for compliance, but how can it feedback into IT, business, and data architecture decisions? How can you use the data lineage to inform data architecture decisions? How would you the catch data elements being pulled from multiple locations?
Data intelligence tools allows for a complete picture of the data architecture landscape which:
However, insights about the data architecture require significant subsequent analysis by IT and business subject matter experts.
With our full Natural Lineage Processing toolkit, many common architectural insights are automated, such as: